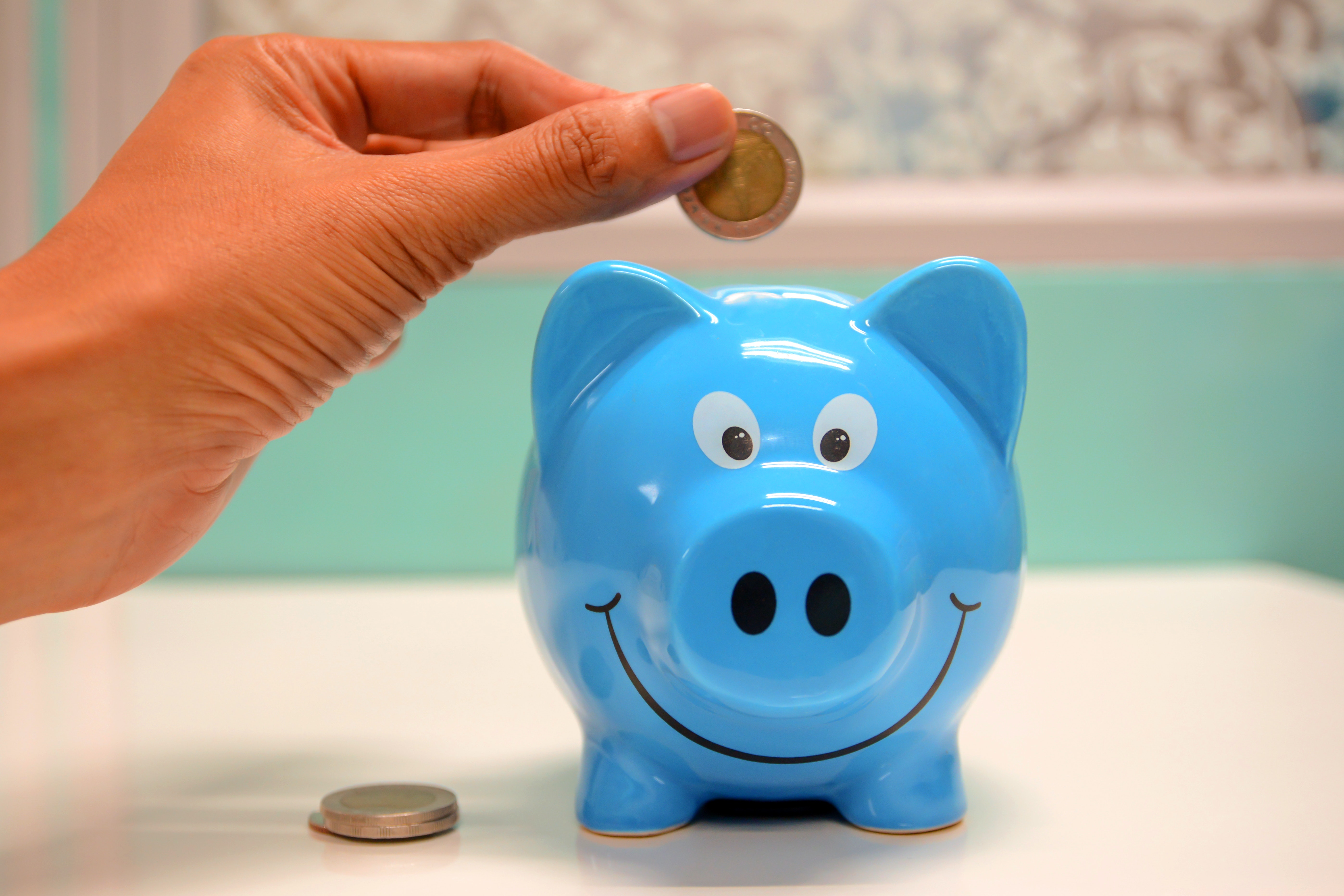
Pig E. Bank Customer Retention Analysis
Project Overview
To increase customer retention, the sales team wants to identify the leading indicators that a customer will leave the bank. Throughout this project, client attributes will be analyzed through data mining in order to discover underlying patterns and determine if specific attributes could indicate whether a customer will leave. This information will then be used to identify the top risk factors that contribute to client loss and modeled in a decision tree.
Limitations
The majority of the data I had access to was fabricated. This made the data prone to biases and generation of unrealistic results.
Although the data was fake, I removed columns that contained customers' personal data to practice ensuring data privacy.
Although the data was fake, I removed columns that contained customers' personal data to practice ensuring data privacy.
Objective
The goal of this project was to introduce me to leveraging Excel and pivot tables for data mining and developing predictive models based on identified patterns.
Tools and Techniques
Dataset was cleaned in Excel. Client attributes were analyzed using pivot tables. Visualizations were created using Excel and PowerPoint.
Data Cleaning
Data cleaning was performed in Excel resulting in a dataset of nearly 1000 records. I assessed the quality of the data by checking for missing values, errors, and inconsistencies. Lastly, I documented all the changes that were made within the dataset during the data cleaning phase.
Descriptive Analysis
Statistical aggregations usually provide a solid initial step to the analysis and help us have an overview of the data. I summarized some of the client attributes through aggregations with the help of pivot tables. From this table, we could already make a few assumptions such as, for example, salary could not be an indicating factor for whether a customer is likely to leave the bank. Some variables, like age in this case, might seem insignificant at first glance since the difference between the average values of two groups might not seem substantial. However, these assumptions require further investigation as these variables might prove otherwise with more in-depth analysis.
.png)
Data Mining: Customer Behavior
In this step, I tried to identify the factors that could potentially increase the likelihood of a customer leaving the bank. Once again, pivot tables came to my aid and enabled me to separate clients into two groups: one for those who have left the bank and a second for those who have stayed. Then, I further segmented clients of both groups based on shared attributes to see what factors could be associated with customer churn.
%20(1).png)
%20(1).png)
%20(1).png)
Data Modeling
Based on the results of the analysis, I ultimately made a decision tree as a predictive model which demonstrates the development of the probability of losing a client based on their demographics and attributes. I started the tree from member activity attribute since it proved to be the most important and leading factor in determining whether a customer will leave the bank.
%20(1).png)
Findings and Recommendations
Out of eight columns that described client attributes, three exhibited a correlation with the likelihood of a client's decision to terminate their association with the bank. The customers who exhibit decreased level of activity, hold only one to two products or services with the bank, and are over 40 years of age are most likely to discontinue their relationship with the bank. The bank should offer enticing promotions and rewards to inactive client to encourage them to maintain their partnership with the bank.